Christopher Beattie
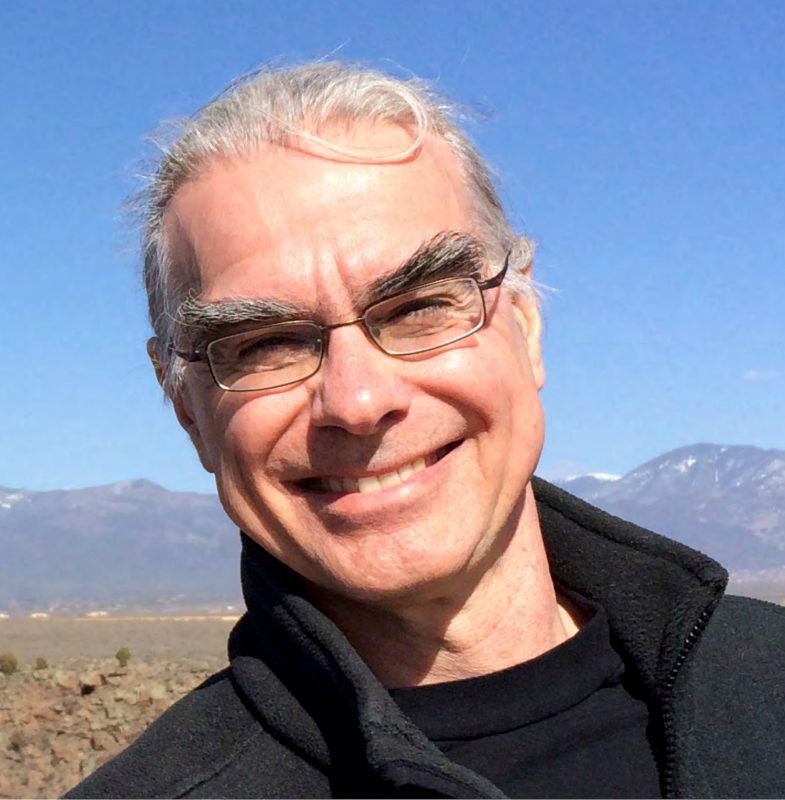
Data & Decision Sciences
Virginia Tech
727 Prices Fork Road
Blacksburg, VA 24061-1026
Professor Beattie's research focus has three principal directions: computational linear algebra, model reduction, and spectral estimation for linear operators.
(1) Computational linear algebra: Computational linear algebra has become a core enabling technology of modern science and engineering - developments in this area are crucial to the development of accurate and efficient computational strategies and not surprisingly, these methods often are at the heart of major computer codes. The development and refinement of these methods to make them fast, accurate, and reliable depends on careful, detailed analysis of their behavior. This has been a central focus of Professor Beattie’s research work with particular emphasis on Krylov subspace methods.
(2) Model reduction: Direct numerical simulation of dynamical systems can play a crucial role in studying a wide variety of complex physical phenomena in areas ranging from ocean circulation to microchip design. Many such phenomena involve heterogeneous mixtures of physical processes that evolve on fine time and length scales while system behaviors of interest occupy much coarser time and length scales. As models are refined at increasingly fine time and length scales in order to attain high accuracy, dynamical systems of enormous scale and complexity are often produced, leading to overwhelming demands made on computational resources. One may address this problem in some cases through methods that encode the fine scale dynamic structure of complex systems into compactly-represented high-fidelity reduced models that may then serve as efficient surrogates for the original systems. While there are a variety of approaches that can accomplish this, Professor Beattie’s principal focus has been on interpolatory methods. This class of approaches is numerically reliable and widely applicable.
(3) Spectral Estimation for Linear Operators: The analysis of physical phenomena often depends on the ability to closely approximate eigenvalues and eigenfunctions of differential operators. Examples range from the prediction of resonant frequencies, vibrational mode shapes, and buckling loads of elastic structures; through the determination of bound state energy levels and associated electronic configurations for atoms and molecules; to the computation of critical values of Reynolds numbers in viscous fluid flows. Eigen problems for differential operators are often fundamentally more difficult than matrix eigen problems due to their infinite dimensional (often noncompact) character.
Professor Beattie has been involved in both theoretical and practical issues involved in the construction of computational methods for approaching problems such as these successfully.